Jump to the content
- {{#headlines}}
- {{title}} {{/headlines}}
The entire world is chock-a-block with AI. It is not only found in smartphones and loudspeakers; it also doses the detergent in washing machines, provides driver assistance features, sorts out spam e-mails and translates texts. Humanity has artificial intelligence to thank for breakthroughs in areas like gene sequencing, which facilitated the development of those efficacious mRNA vaccines in the fight against the Covid pandemic. In some areas of medicine, humans and AI work hand-in-hand – in breast cancer screening in radiology, for example. Here, the findings are assessed according to the four-eyes principle: the images are examined separately by two individuals. It is now often artificial intelligence that takes on the role of the second assessor. With the aid, amongst other things, of artificial neural networks, the computer scientist, Daniel Rückert, has significantly improved the quality of medical imaging. The Alexander von Humboldt Professor for AI at the Technical University of Munich is convinced that the strengths of AI and those of humans complement each other ideally. “Of course, humans have the advantage that they can interpret images correctly even if they don’t look like the ones they trained with. On the other hand, people make mistakes, for example when they are tired. The huge advantage of machine learning or AI models is that they always give you an answer, irrespective of the number of images you show them. So, if you get humans and AI to work together you can combine the best of both worlds and, hopefully, eliminate the respective disadvantages.”
A world
teeming with AI




An ingenious move flabbergasts the Go community
But AI is not only capable of supporting people. In certain areas it is now beginning to successfully compete with them. One historic example was the victory of AI in the strategically complex board game Go. In March 2016, one of the world’s best Go players, the South Korean Lee Sedol, lost four of his five matches against the computer programme, AlphaGo. It was the 37th move in the second match that was to mark a new milestone in machine intelligence. Commentators couldn’t believe their eyes. It looked as though someone had clicked on the wrong button of an online game. At that point, the world-class player Lee Sedol seems to have intuited the implications of the move. He left the room for a few minutes.
No top-rank player had ever performed a comparable move in the board game. So, AlphaGo’s artificial intelligence could not have witnessed a move like that before. The computer had not simply replicated something that had been programmed in; it had applied its knowledge about the game intelligently.
How does a computer manage something like that? Classic AI is based on rules and symbols and functions well in predictable environments. It adheres to decision trees or searches for solutions from a set quantity of potential solutions. Everything it knows about the world has been fed into it by humans. Modern AI of the type used in AlphaGo, on the other hand, is effectively based on our brain. Neurons that are connected in our brain and sometimes fire and sometimes don’t are reproduced digitally. They respond to different stimuli. “These digital neurons have one thing in common with the brain. They are connected to other neurons. And whether they ‘fire’, depends on the amount of input they get. One neuron fires at the next one according to a mathematical formula which tries to reproduce what’s taking place amongst the neurons in the brain,” the Humboldtian Christian Becker-Asano, Professor of Artificial Intelligence at the Hochschule der Medien in Stuttgart, explains.
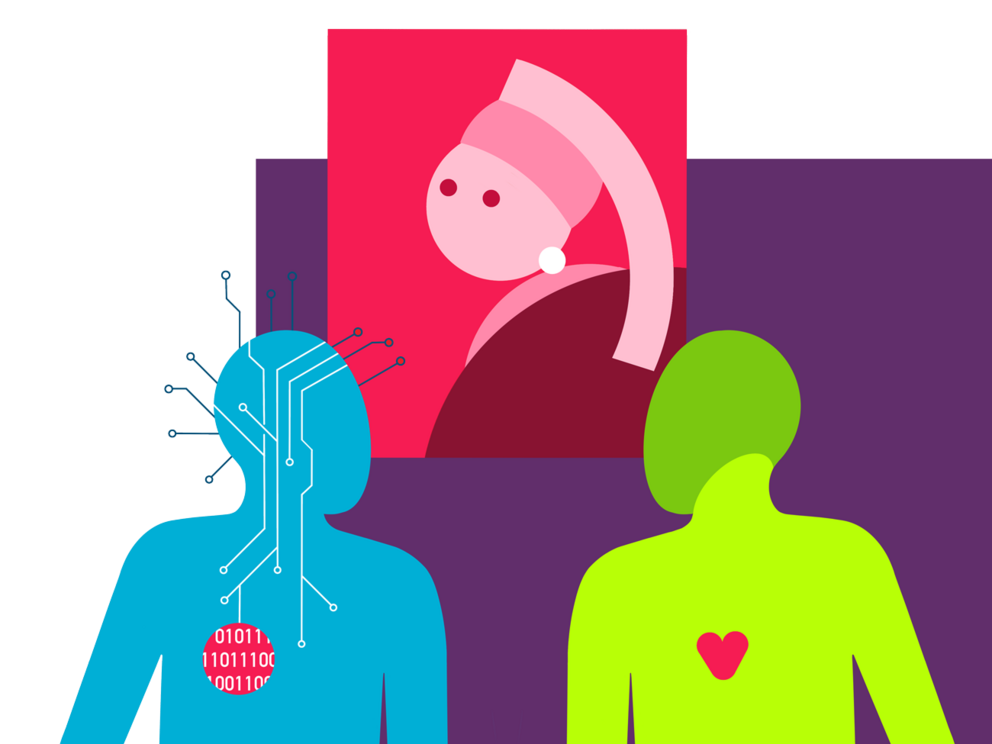
But even if artificial intelligence were one day able to function like human intelligence, if it could perceive the world the way we do, it would probably still be lacking something crucial: an emotional relationship with whatever it perceives. The Humboldtian Tobias Matzner, professor in the Department of Media, Algorithms and Society at Paderborn University, describes the difference between humans and machines: “An algorithm looking at an image simply sees rows of pixels. Nothing else. And for an algorithm, these pixels ‘equal image’, irrespective of whether the image is noisy or whether it shows a friend, or a dog, or just something blurry. When we look at an image, it immediately triggers a raft of associations.” That is why AI needs far more examples to learn something new than humans do.
The essence of human conversation is our ability to recognise and respond to emotions.
Milica Gašić therefore wants to humanise the way AI learns. The Sofja Kovaleskaja Award Winner at Heinrich Heine University Düsseldorf takes her inspiration from the way animals and children learn. “I would like to build systems that continue developing over time as humans do. Every day, I see how my little daughter learns new things, and we really have a fantastic ability to pick up new things and to know what to do with them,” says Gašić. Her aim is to improve language systems so that we can talk to AI just as we do to other people. So far, it is not just a more eloquent use of language that machines lack. “We shouldn’t forget what the essence of human conversation is: above all, our emotions and our ability to recognise and respond to emotions,” Gašić emphasises. She wants to discover how machines’ language competence can be improved to a level that means they can even be used in psychological consultations. Emotional empathy plays a role in this. If a robot could feel pain, perhaps it would treat people with greater empathy.
What goes on inside the black box AI?
Mutual trust is one of the prerequisites for free and open communication between people. Here, too, AI has some catching up to do. News about fatal accidents caused by selfdriving, AI-controlled cars or popular science fiction themes like the evil AI striving for world domination make people uneasy. In order to build trust, it would be helpful to understand how AI thinks, how it makes assessments and decisions.
But that is not so easy. Most modern AI systems are black box models. They receive input und deliver output. They recognise a dog or a cat, a stop sign or a speed limit, a tumour or a rare disease. But how they do it is their own well-kept secret.
We don’t understand why mysterious mistakes keep occurring because we don’t know what the algorithm really is doing inside.
“Neural networks are impenetrable,” says Daniel Rückert. “If we want to automate measuring procedures, we can use the measurements on the screen to show the radiologist how the computer has calculated the volume of the tumour. The radiologist sees it all, too, and can judge whether it’s right or not. We don’t need to explain exactly how we delineated the tumour. Where it starts to get tricky, however, is when you want to use the results of your AI model to formulate hypotheses on how a disease will develop, for example, or what the origins of the disease were.”
Christian Becker-Asano sometimes worries that some scientists are perfectly satisfied when something works without understanding what is going on in the background. This leads to AIs that do normally work, but in some situations suddenly don’t. “We have great achievements in practical applications with some very mysterious mistakes that the machines seem to make if there’s some noise in the image. We don’t understand why because we don’t know what the algorithm really is doing inside,” says Becker-Asano.

Humans can recognise a stop sign even when the image is noisy, or the colours are wrong. But AI can get confused even by just a sticker on the stop sign, or weather and light conditions that are different from the ones in the test environment.
“When a mistake does happen and the car fails to stop at a stop sign because the AI has classified something wrongly, for instance, theoretically we could then analyse the machine’s memory. There are masses of data on the computer, and you can take a snapshot of the neural network at the very moment the machine makes the mistake. But all you discover is a load of data,” says Becker-Asano, explaining the problem. Even simply adding further training data does not guarantee that something that worked before will work again in the future, Daniel Rückert emphasises. “ Precisely because we don’t exactly know what’s going on inside the black box.”
According to Tobias Matzner, making the black box transparent would thus be an important step in AI development so that those who use AI can trust it. It is important to him that people understand what happens to their data when they use artificial intelligence and that they are told how their data can influence algorithms’ decisions. “Imagine you are applying for a job. An algorithm rejects your application. Then you’re not really interested in how the algorithm works, instead you ask yourself what would have to be different about you for you to get the job.”
AI learns discrimination
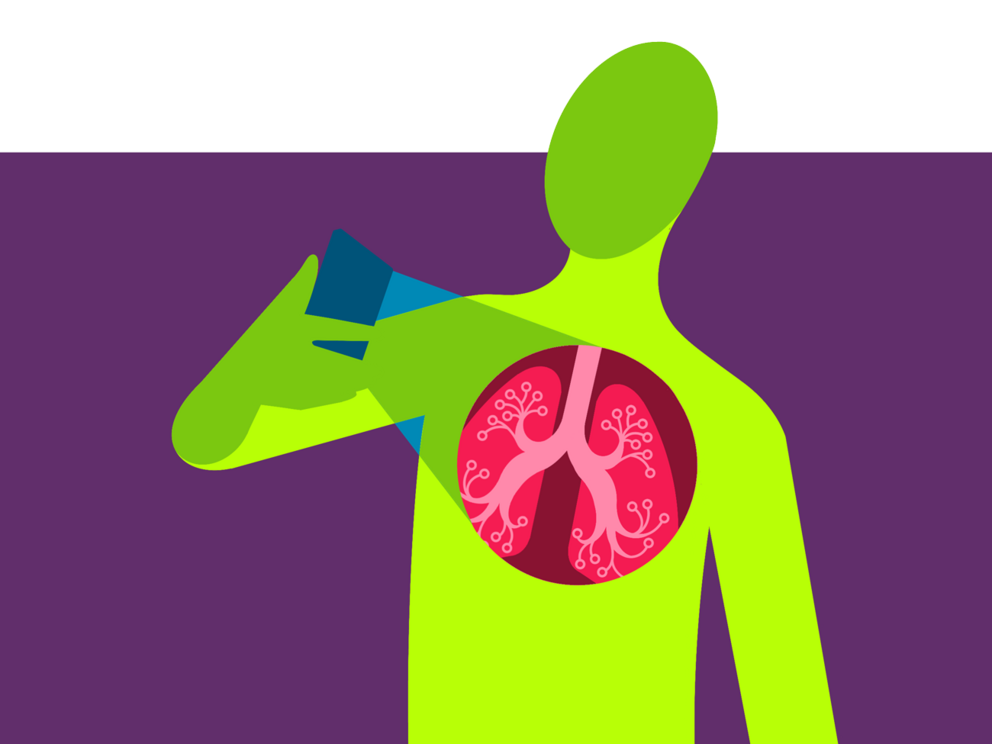
This example was not plucked out of thin air. A major global corporation really did develop AI that was supposed to help select job applicants. Professor Aimee van Wynsberghe of the University of Bonn describes this as a case of discrimination by AI: “They used ten years of historical data to create a recruiting tool. When those responsible were then going through the CVs to choose candidates for the positions, they found that the machine was only recommending men for the managerial positions, never women.” The explanation is that existing inequalities had found their way into the training data which the AI had then adopted. Aimee van Wynsberghe suggests using this downside of AI to advantage: “If you use this recruitment tool to investigate discrimination in corporate culture instead of as a basis for recruiting new staff, it’s a fascinating tool. That is how the technology sheds light on certain forms of inequality. And then we have a choice: do we perpetuate these systems of inequality, or do we stop and make a difference right now? AI has enormous potential for our society, but it’s up to us how we decide to use it.”